by James Benco
ABSTRACT
An important aspect of life and governance is risk assessment. Controlling floodwaters, minimizing earthquake damage, shoring up landslide prone slopes, are all examples of our modern society minimizing risk from the harms of natural disasters. Here in in Illinois, many of the threats mentioned are of minimal risk, landslides are nearly non-existent and all earthquakes are typically sub magnitude 2.6. The natural threats that pose a risk to Illinois are floods in the Mississippi river, and storm events. These storm events can become tornadoes if the right criteria are met. This paper seeks to act as a support in the risk assessment of this natural hazard in Illinois. This will be done with a stochastic model of tornado-genesis for the state of Illinois. This risk assessment will be at the county level, and as such will be most useful for those county governments and state governments to direct funding and material. Risk as seen through this model have shown that certain counties such as Logan, Christian and Mercer counties contain the largest tornado risk.
- INTRODUCTION
- Purpose and Scope
The main objective of this study is to determine which counties within Illinois have the greatest tornado risk, and to assess the magnitude of that risk. By applying a stochastic model with simulated and observed data, we can ascertain with reasonable error the primary counties of tornado activity in the past 20 years.
- Importance of This Study
This is an important question; tornadoes are a powerful force of nature and rebuilding from an event can take considerable resources and time. Any loss of infrastructure functionality for a community can significantly affect the economic vitality, and result in social disruption and depopulation (Hassan, 2017). Being able to already have resources nearby in case of an event, on the county level can greatly alleviate the stresses on a community. This is how this study can help this situation, as efficient use of resources stationed in the correct counties can best use the resources available to help the greatest amount of people.
- LITERATURE REVIEW
2.1 Overview
With the importance of this atmospheric setting, strategies to precisely determine the risk to counties are paramount. This study will cover the tornado risk to Illinois counties by using a stochastic model to result in probability scores, based on the past 20 years of atmospheric data from NOAA.
2.2 Tornado Genesis
In order to fully understand tornado risk assessment, a fundamental understanding of tornado-genesis is in order. Tornado-genesis it the creation of a tornado, these are through super-cell thunderstorms, although a significant fraction of tornadoes are associated with nonsupercellular convection, rough estimates for this tornadogenesis are up to 20% (Trapp et al., 2005a). Tornadoes are formed as the extension of rotation from the mesocyclone of the supercell. In particular needing the rear-flank downdraft of the supercell to be buoyant enough to have positive buoyancy when reaching the ground level, thus able to be spun back up through the mesocyclone updraft (Markowski & Richardson, 2009). The stages of tornadogenesis are shown in figure 1 below.
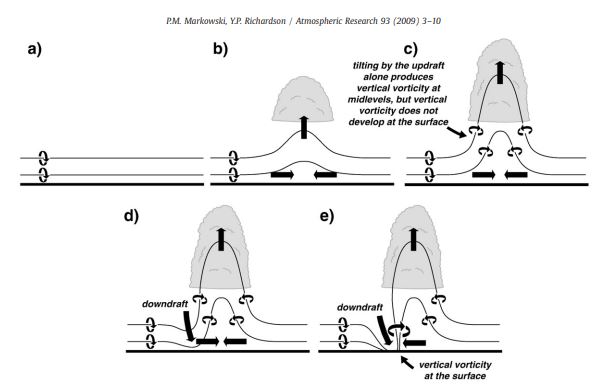
Figure 1. In this figure one can see the stages of uplift of the supercell from stage a being ambient conditions, with natural cycling of the airmass. Stage b shows the first signs of updraft due to the ground-level air mass having positive buoyancy relative the to the overlaying air mass, this causes the cycling air to partially rotate vertically and the lower air mass contract. Stage c can see the full tilting of the updraft producing the vertical vorticity which is the mesocyclone of the supercell. Stage d references the rear-flank downdraft bringing colder less buoyant air from the top of the cell to the surface, along with the surface contraction. Stage e occurs when that rear-flank downdraft although less cold and less buoyant, is still buoyant enough to return upwards through the updraft, in which case the vorticity elongates and tightens from the mesocyclone to the surface, which is called a tornado. Modified from Markowski & Richardson 2009.
The United States has the most tornadoes in the world each year. This is due to a few unique factors that lend itself to supercell thunderstorm creation. One of the largest factors is having northward movement of moist air masses from the Gulf of Mexico (Boruf et al.,2003). The other factor is the elevated mixed-layer (EML) inversion over the Great Plains area. This is a dry air-mass that comes eastward from the Rockies and other elevated regions in the west, producing a system in which moist warm buoyant air is overset by a dry cold air mass, causing an inversion. Once a storm is capable of moving upwards through the EML in a region, that storm will be able to rapidly expand and become a supercell event (Lanicci et al., 1990).
2.3 Primary Technical Approaches
Stochastic modeling was used to simulate observed tornado generation frequencies and the location of the tornados. The workflow of the model is presented below in Figure 2.
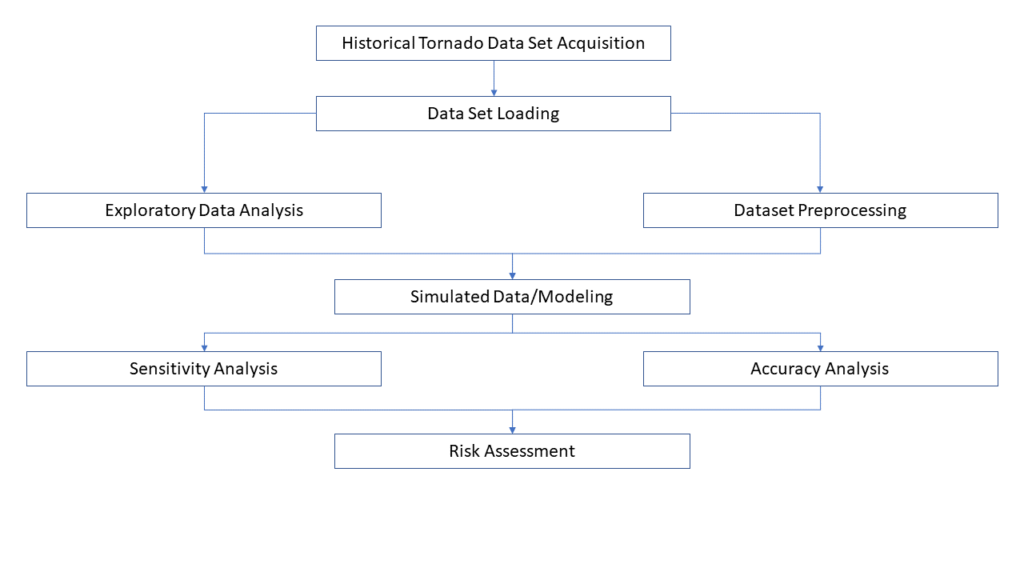
Figure 2. Depicting the workflow of the stochastic model on a general level for this study. It is important to note that although data came from NOAA for a specific selection, incomplete/duplicated entries were included in the original data file, these have been removed for clarity.
2.3.1 Stochastic Model
The stochastic model used for this dataset was based on Fan and Pang Genesis model for the United States as a whole. The Probability Density Function (PDF) used in this study was generated from the normal distribution with mean and standard deviation derived from the observed data. The simulated data was then compared to the observed data through Probability Mass Function of the two datasets and plotted on overlayed Q-Q plots for accuracy.
- DATA AND PREPROCESSING
3.1 Data Source
Data for this study comes from the NOAA Storm Events Database. Search parameters include: Event Types: Tornado, All counties in Illinois. The search was limited to 500 records due to the size of the selection, however this is a sufficient dataset for the scope of this study. Data used for this study is located in Appendix A.
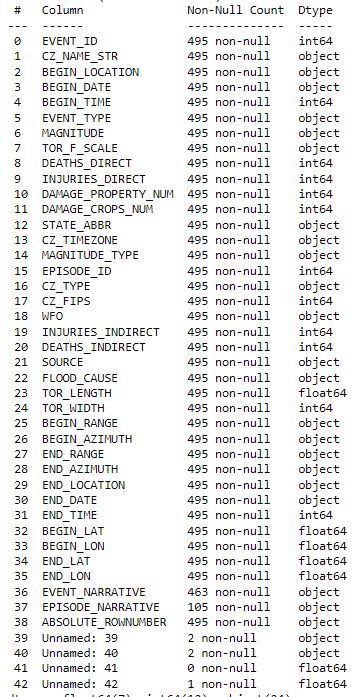
Figure 3. Displays the column names of information gathered from the NOAA Storm Events Database. For the scope of this study only location, events and time data were used. However, further studies would be able to incorporate track modeling and tornado hazard analysis in comparison with existing population centers and structures.
3.2 Data Preprocessing
Data preprocessing took form of removing all NaN values and entries with missing location, or time data. This changed our initial search result from 500 to 495 events. Data was then processed into new dataframees grouped-by year and county. This grouped data is the observed dataset for this study.
- METHODOLOGY
4.1 Exploratory Data Analysis
The initial exploratory data analysis showed important information about the dataset. In figure 4 displaying the results of events from 20 years listed by county.
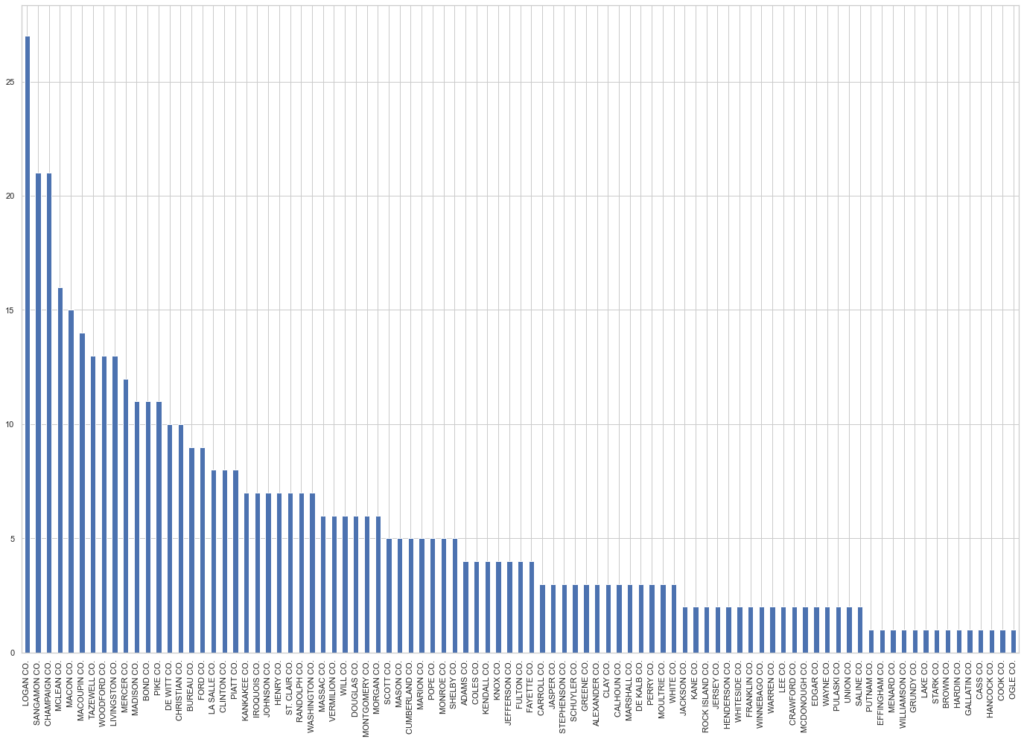
Figure 4. This graph shows the total events from the dataset listed by county. This shows that Logan, Sangamon and Champaign counties have experienced the most events in the past 20 years. The data distribution resembles part of a normal distribution.
Figure 5 displays the location information on a township level for all the events in the database. Most townships in the study only experience one (1) event in the past 20 years, and as such a study of increased resolution is not feasible from this dataset.
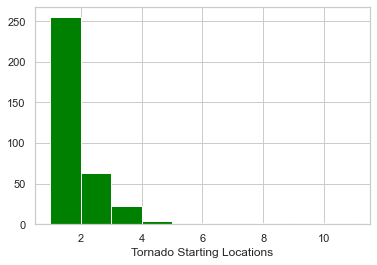
Figure 5. This figure displays tornado event data per township. Noting that most townships experienced one event in the past twenty years.
4.2 Genesis Model
The model used in this study simulated data using a random normal distribution of the probability density function based off the mean and standard deviation of the observed data.

Figure 6. This displays the function used to generate the simulated data and the parameters used in that model generation. The mean and standard deviation used was based off of the observed data’s probability density function (PDF) mean and standard deviation.
- RESULTS
Results from this study show the distribution of events across county and year. Figure 7 displays the yearly events for each county.
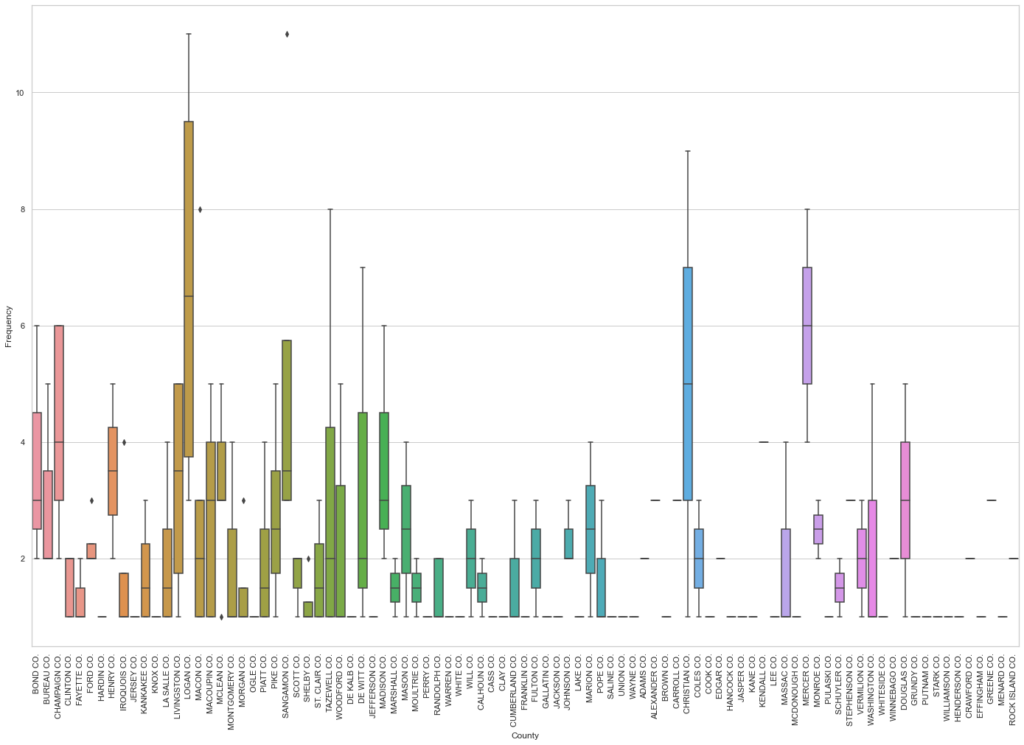
Figure 7. Displays the events per county per year. Showing that the Logan co has the greatest average amount of events on average, but with large variability each year. The entire dataset has large variability which increases difficulty in modeling that data. From this the counties at the highest risk are Champaign Co, Christian Co, and Mercer Co.
Demonstrating the accuracy of the model data, we first will compare the model results against the observed results, as presented in Q-Q plot in Figure 8 below.
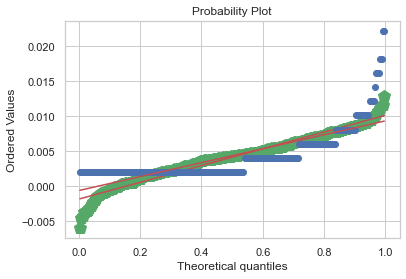
Figure 8. Displays the results of the PDF of the observed and simulated datasets. The observed dataset (blue) and the simulated dataset (green). This Q-Q plot shows that both datasets are normally distributed and of similar shape. There are differences in the distribution, however the differences are within reasonable correlation to be useful.
Accuracy of the model was determined through spearman correlation coefficients between the two datasets. The spearman correlation coefficient was 0.01555 with a p-value of 0.814. The p-value of the correlation is an acceptable amount of error, with a positive correlation coefficient representing a positive relationship between the two datasets.
5.1 Sensitivity Analysis
Sensitivity analysis was performed on the model to determine the importance of model parameters. The mean and standard deviation of the normal distribution were increased by 10% and compared to the observed dataset.
Data | Covariance | Pearson Correlation | Spearman Correlation | P-Value |
Model | -1.3432e-05 | 0.5695 | 0.01555 | 0.814 |
Model +10% Mean | 1.3432e-05 | 0.4722 | -0.06127 | 0.355 |
Model +10% STD | 1.3432e-05 | 0.7743 | -0.04825 | 0.4665 |
Table 1. This table compares accuracy metrics of the model and subsequent models as compared to the original observed dataset. Models were adjusted by 10% increase in the mean of the normal distribution, and the standard deviation of the normal distribution.
- Discussion
6.2 Model Discussion
The genesis model was from a normal distribution based on the nationwide Genesis model from Fan and Ping 2019. In that study simulated data was generated from a negative binomial distribution and in our study using a normal distribution. The equation this study used as a starting point is presented below:

Figure 9. This is the negative binomial distribution used to create the Genesis model for Fan and Ping Stochastic Model. NTor is the number of tornadoes which occurred in a given area in a year, p = 0.0253 and r = 31.065.
6.3 Unaccounted Effects Discussion
This model was a relatively simplistic view of the tornado risk posed to Illinois. If one were to increase the accuracy of the model, incorporating specific regional traits that affect tornadogenesis would be necessary. One such trait is the effect of Urban Spawl on tornado-genesis and impact. The urban heat island effect is one that simply by the concentration of human activities, industries and energy consumption in a dense area creates a heat island as compared to the surrounding air masses. This heat island, helps suppress or dissipate small tornadoes near the interior of the heat island but concentrates them along its boundaries (Fujita 1973a). Fujita, 1973a has noted that the distribution of tornadoes around Chicago have a horseshoe shape with the open end on Lake Michigan. A more accurate model would incorporate this into the simulation, as the city of Chicago suburbs expand and as such the heat island expands as well.
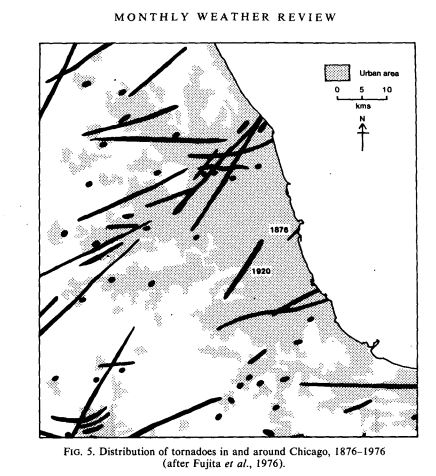
Figure 10: This map of tornado distribution around the city of Chicago (gray shaded regions) show that the central most dense sections of the city experienced the least number of tornadoes. Only two events (1876,1920) were in the dense central portion of the heat island. In regard to the 1876 tornado there is doubt this is a true tornado and not instead a land spout which is formed from different atmospheric processes. Most tornadoes instead clustered around the borders of the inferred heat island and demonstrate the effect of a strong heat island on tornadogenesis.
6.4 Original Implementation and Problems Discussion
Originally this study was to include a chloropleth or heat map of PMF values from our observed data and simulated data. Unfortunately, the primary method to do this in python is through the geopandas library and I was unable to properly install this library in my conda environment, or pip install this library directly into the notebook. The problem lies with the Fiona package, as I was not able to resolve this in time, the geographic map of this study’s PMF values were not able to be plotted and compared visually.
- CONCLUSIONS
This study modeled tornado risk in Illinois counties using tornado events data with a model using a normal distribution based on the observed data. This is useful as this model can be used to allocate resources to their most useful counties. The stochastic model was based on the NOAA Storm Events data for counties in Illinois.
Conclusions from this study include:
- The counties that have the highest tornado risk include: Logan Co, Christian Co, and Mercer Co. and that relief resources should be concentrated in these counties.
- Most tornado events are clustered in county resolution, however not clustered in township resolution.
- Tornado event risk by county in Illinois can be modelled by a normal distribution.
- FUTURE WORK
This is an important problem to be solved as any increase in efficient use of resources can translate into greater increase in positive outcomes for the community. Future steps to improve upon this study include:
Changing the methodology of the study to a Generative Adversarial Neural Network instead of a stochastic model. This would provide a much more predictive tool to see how risk of the region is shifting from historical trends.
Incorporating a Track Model in addition to the Genesis Model, to create Tornado Hazard maps of Illinois. Thus, combining city locations and the probability of a tornado moving through cities and towns.
Applying regional effects to tornado genesis such as the urban heat island effect, lake breeze effect and increased regional moisture content around the Mississippi river.
8. FUTURE WORK
This is an important problem to be solved as any increase in efficient use of resources can translate into greater increase in positive outcomes for the community. Future steps to improve upon this study include:
- Incorporating a Track Model in addition to the Genesis Model, to create Tornado Hazard maps of Illinois. Thus, combining city locations and the probability of a tornado moving through cities and towns.
- Applying regional effects to tornado genesis such as the urban heat island effect, lake breeze effect and increased regional moisture content around the Mississippi river.
- Changing the methodology of the study to a Generative Adversarial Neural Network instead of a stochastic model. This would provide a much more predictive tool to see how risk of the region is shifting from historical trends.